GUI-World Team
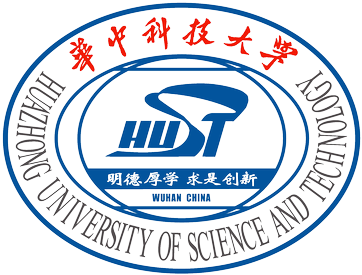
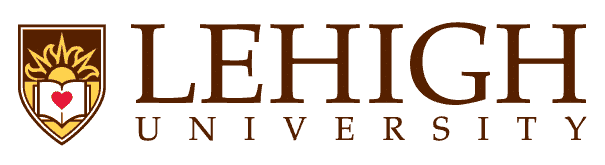
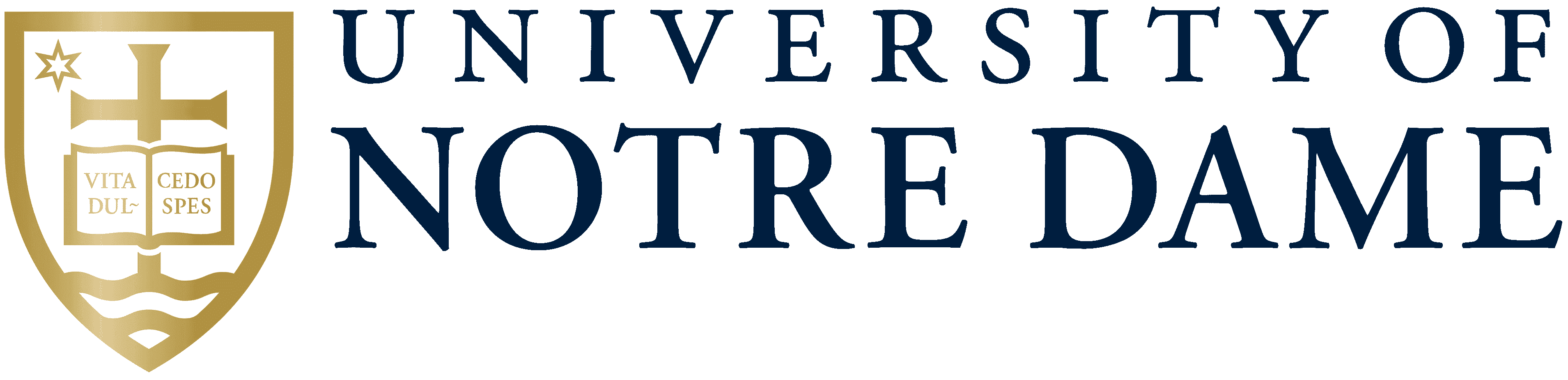
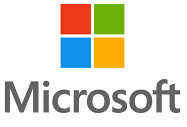
GUI-WORLD | |
---|---|
Instances | 12,379 |
Sem. | Both |
VL | ✔️ |
Video | ✔️ |
Web | ✔️ |
Mob. | ✔️ |
Desk. | ✔️ |
XR | ✔️ |
Sequential | ✔️ |
CrossApp | ✔️ |
Dynamic | ✔️ |
Detailed Tasks | GUI Understanding Instruction Following |
AgentStudio | OSWorld | UGIF | AitW | Mind2Web | Rico | FerretUI | WebArena | MetaGUI | MiniWoB++ | OmniAct | MMINA |
---|---|---|---|---|---|---|---|---|---|---|---|
304 | 369 | 523 | 715,142 | 2,350 | 72,219 | 123,702 | 812 | 1,125 | 100 | 9,802 | 1,050 |
High | High | High | High | Both | Low | Low | Low | Low | Low | Low | Low |
✔️ | ✔️ | ❌ | ❌ | ✔️ | ✔️ | ✔️ | ❌ | ❌ | ❌ | ✔️ | ✔️ |
✔️ | ✔️ | ❌ | ❌ | ✔️ | ✔️ | ❌ | ❌ | ❌ | ❌ | ❌ | ✔️ |
✔️ | ✔️ | ❌ | ✔️ | ✔️ | ❌ | ✔️ | ✔️ | ✔️ | ✔️ | ✔️ | ✔️ |
❌ | ❌ | ✔️ | ❌ | ❌ | ✔️ | ✔️ | ✔️ | ✔️ | ✔️ | ❌ | ✔️ |
❌ | ❌ | ❌ | ❌ | ❌ | ❌ | ✔️ | ❌ | ❌ | ❌ | ✔️ | ❌ |
❌ | ✔️ | ❌ | ❌ | ❌ | ❌ | ❌ | ❌ | ❌ | ❌ | ❌ | ❌ |
✔️ | ✔️ | ✔️ | ✔️ | ✔️ | ✔️ | ✔️ | ✔️ | ✔️ | ✔️ | ✔️ | ✔️ |
✔️ | ✔️ | ❌ | ✔️ | ✔️ | ✔️ | ✔️ | ✔️ | ✔️ | ❌ | ✔️ | ✔️ |
❌ | ❌ | ❌ | ❌ | ❌ | ❌ | ❌ | ❌ | ❌ | ❌ | ❌ | ❌ |
General Control | General Control | UI Grounded Instruction Following | GUI Understanding | Web Navigation | UI Code/Layout Generation | UI Grounding & Understanding | Web Navigation | Mobile Navigation | Web Navigation | Code Generation | Web Navigation |
Setting | F.K. | E.K. | Data | Software | Website | XR | Multi | IOS | Android | Avg. | ||||||||
---|---|---|---|---|---|---|---|---|---|---|---|---|---|---|---|---|---|---|
I. | V. | MC | Free | MC | Free | MC | Free | MC | Free | MC | Free | MC | Free | MC | Free | |||
Baseline | - | 8 | - | - | 45.5% | 2.144 | 42.6% | 2.221 | 44.0% | 2.005 | 40.4% | 2.222 | 40.2% | 2.169 | 44.7% | 2.119 | 42.9% | 2.147 |
- | 16 | - | - | 45.1% | 2.144 | 41.8% | 2.240 | 41.0% | 2.007 | 40.7% | 2.238 | 39.9% | 2.138 | 44.7% | 2.147 | 42.2% | 2.154 | |
GUI-Vid | 8 | 8 | ✖ | ✔ | 58.3% | 2.709 | 53.6% | 2.817 | 62.2% | 2.626 | 54.2% | 2.627 | 53.1% | 2.708 | 54.9% | 2.501 | 56.0% | 2.665 |
✔ | ✔ | 59.9% | 2.856 | 54.1% | 2.925 | 59.0% | 2.751 | 52.1% | 2.837 | 50.0% | 2.756 | 54.0% | 2.571 | 54.8% | 2.782 | |||
16 | ✖ | ✔ | 59.0% | 2.709 | 55.1% | 2.821 | 62.8% | 2.645 | 53.3% | 2.624 | 55.5% | 2.727 | 55.7% | 2.501 | 56.9% | 2.671 | ||
✔ | ✔ | 59.9% | 2.847 | 54.1% | 2.957 | 55.6% | 2.764 | 52.9% | 2.861 | 51.8% | 2.772 | 53.4% | 2.572 | 54.6% | 2.796 |
GPT-4V and Gemini excel in common scenarios such as mobile and website interfaces but show marked deficiencies in more complex GUI environments like XR and multi-window interactions, across both captioning and intricate tasks. This performance gap highlights a significant shortfall in understanding environments where GUI elements are scattered and demand sophisticated interpretation. It emphasizes the critical need for specialized benchmarks and datasets tailored to these complex GUI scenarios, which is essential for enhancing the GUI-oriented capabilities of MLLMs, paving the way for them to become truly reliable and high-performing general control agents.
Across both basic tasks such as captioning and more complex tasks like prediction and reasoning, significant variations are evident among keyframe selection methods. GPT-4V and Gemini significantly benefit from using random-selected and human-selected keyframes, scoring approximately 0.2-0.3 points higher in both captioning and free-form tasks than those using programmatic extraction. This suggests that traditional keyframe technologies, designed for natural videos, are less effective for detecting essential GUI operations, particularly when subtle movements like mouse clicks and dynamic changes are involved. Conversely, the difference in performance is relatively smaller in Qwen-VL-Max, indicating that while keyframe selection methods are crucial for models proficient in GUI content, they exert less influence on less capable models.
In the fine-grained tasks, GPT-4V and GPT-4o excel with static GUI content and prediction tasks over image sequences but struggle with providing detailed descriptions for entire videos and dynamic GUI content. This discrepancy is attributed to minor variations in GUI that significantly impact descriptions. Enhancing the number of keyframes and the granularity of perception might mitigate these issues. Among VideoLLMs, ChatUnivi excels in conversational tasks by effectively leveraging contextual nuances, particularly in subsequent rounds, yet it underperforms in GUI-oriented captioning tasks. In contrast, GUI-Vid demonstrates proficiency in sequential tasks but falls short in both captioning and static content handling. This gap is linked to deficiencies in GUI-Vid’s pretraining, which lacked comprehensive GUI content crucial for effective vision-text alignment, as evidenced by its poor performance and an instruction tuning process also failed to fully address these shortcomings.
Integrating detailed textual information slightly outperforms purely vision-based inputs or detailed captions, akin to a Chain of Thought (CoT) setting. Surprisingly, GPT-4V excels in caption and prediction tasks with just detailed captions, providing insights on enhancing specific GUI-oriented tasks through additional textual information. However, it still falls short in more challenging tasks, such as retrieving static or dynamic content. This underscores the critical role of visual perception in GUI environments, where even minor changes can significantly impact outcomes.
As a pioneering study in training VideoLLMs as screen agents, GUI-Vid significantly outperforms the baseline model, showing an average improvement of 30% across various tasks and GUI scenarios, even surpassing the commercial ImageLLM, Qwen-VL-Max. This enhancement is particularly notable in captioning and prediction over image sequences, where GUI-Vid matches the performance of GPT-4V and Gemini-Pro. Our two-stage progressive fintuning significantly enhances the performance in all GUI scenarios. Remarkably, GUI-Vid scored 3.747 in caption tasks within the XR scenario, highlighting its potential in XR applications and the high-quality annotations provided by our dataset. However, in Multiple-Choice QA and Chatbot tasks, GUI-Vid still lags behind industry leaders like GPT-4V and Gemini-Pro, a discrepancy likely due to the baseline LLM’s weaker performance and the challenges of instruction-based fine-tuning.
Our two ablation studies during the fine-tuning phase demonstrate that utilizing GUI image-text captioning data significantly enhances the model's preliminary understanding of GUI elements, outperforming training that relies solely on videos. Additionally, an increased number of keyframes correlates with improved performance across various scenarios, notably in environments featuring multiple windows and software applications. Further evidence reveals that higher image resolutions substantially boost task performance, both basic and complex, for GPT-4o. These findings underscore the potential for further developing a more robust GUI Agent.
@misc{chen2024guiworld,
title={GUI-WORLD: A Dataset for GUI-oriented Multimodal LLM-based Agents},
author={Dongping Chen and Yue Huang and Siyuan Wu and Jingyu Tang and Liuyi Chen and Yilin Bai and Zhigang He and Chenlong Wang and Huichi Zhou and Yiqiang Li and Tianshuo Zhou and Yue Yu and Chujie Gao and Qihui Zhang and Yi Gui and Zhen Li and Yao Wan and Pan Zhou and Jianfeng Gao and Lichao Sun},
year={2024},
eprint={2406.10819},
}